Research on tensor signal processing is crucial in the field of wireless communications due to the increasing complexity and dimensionality of data encountered in modern systems. Traditional signal processing techniques often do not effectively exploit the multi-dimensional nature of data and channels, such as those generated by sensor arrays or multiple-input multiple-output (MIMO) systems. Tensor decompositions provide a powerful framework for interpreting, analyzing, and estimating multi-dimensional signals and channels, improving performance in tasks like channel estimation, multi-user separation, and beamforming, to mention a few. By leveraging tensor decomposition methods, we can extract relevant parameters from high-dimensional noisy data, enhancing detection and improved communication reliability. Moreover, by integrating machine learning techniques, tensor signal processing offers new avenues for adaptive communication systems that can learn from and respond to dynamic environments, ensuring robust system performance in specific scenarios.
Click below to listen to a high-level and intuitive conversation on the subject!

Tensor decompositions & algorithms

The Role of Tensor Signal Processing in Wireless Communications
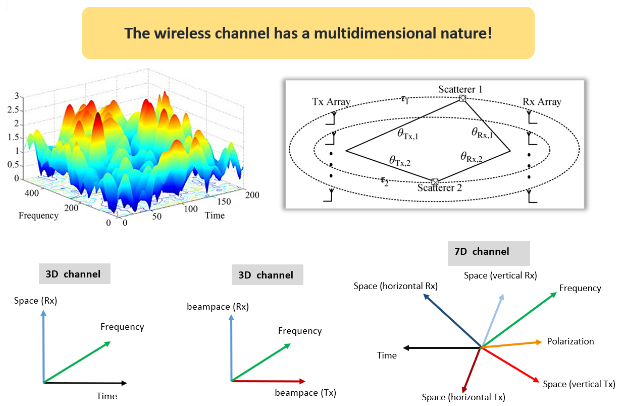
Tensor decompositions for wireless channel modeling & estimation

Tensor decompositions and reconfigurable intelligent surfaces (RIS)

Tensor models for space-time-frequency processing in MIMO systems

Tensor decompositions for MIMO relaying systems

Tensor decompositions for multilinear filtering